Safeguarding Data Privacy: The Advancement of Private Large Language Models (LLMs)
The world of artificial intelligence has witnessed remarkable progress, with Large Language Models (LLMs) emerging as one of its most transformative innovations. Powered by Natural Language Processing (NLP), LLMs have revolutionized how we interact with technology. However, concerns regarding data privacy and security have given rise to a new trend - developing and adopting private Large Language Models. In this article, we delve into the significance of private LLMs and how they address the mounting apprehensions surrounding data protection.
Understanding Large Language Models (LLMs):
LLMs are state-of-the-art AI models designed to understand and generate human-like language. They have demonstrated exceptional capabilities in various language-related tasks, such as language translation, text summarization, question-answering systems, and even creative writing. Some prominent examples include OpenAI's GPT (Generative Pre-trained Transformer) series and Google's BERT (Bidirectional Encoder Representations from Transformers).
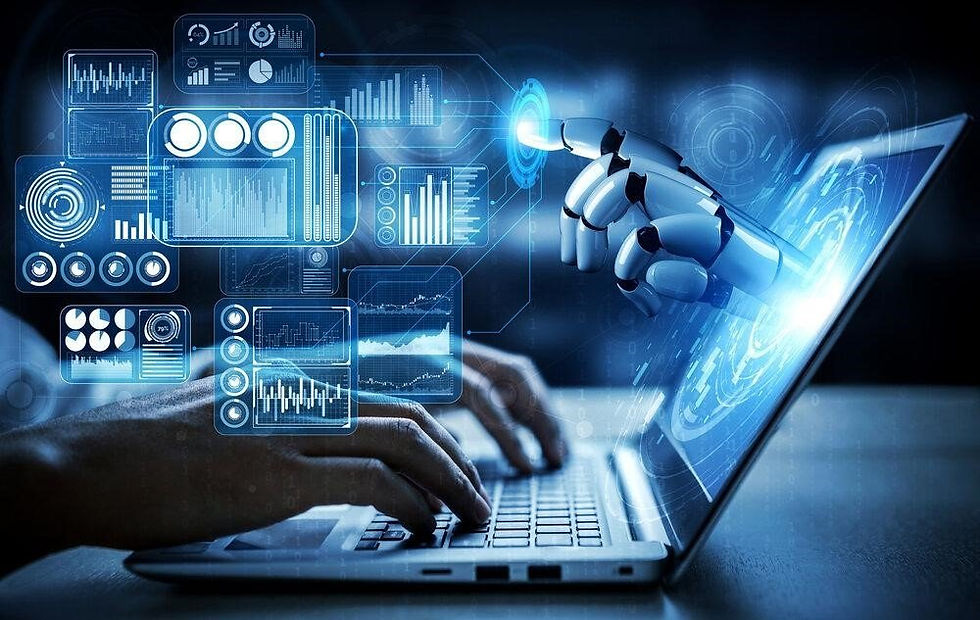
The Predicament of Privacy:
Despite their impressive achievements, LLMs have encountered privacy concerns due to their reliance on extensive datasets, which may include sensitive user information. This accumulation of data raises fears of potential privacy breaches and unauthorized access to personal data, prompting the need for more secure approaches.
The Emergence of Private LLMs:
In response to these concerns, researchers and developers have been diligently working on creating private LLM. These models prioritize data privacy by employing various techniques to protect user information while still providing effective language processing capabilities.
Federated Learning: One approach involves federated learning, where the LLM is trained across multiple devices or servers, each having access only to a portion of the data. The model aggregates knowledge from these decentralized sources without directly accessing individual data points, ensuring privacy is maintained.
Differential Privacy: Another essential aspect is differential privacy, which introduces controlled noise to the training data, making it difficult for the model to memorize specific details about any individual data sample. This protection prevents the inadvertent capture of sensitive information during training.
Encrypted Computation: Encryption techniques are employed to process data in an encrypted form, ensuring that even during computation, no unencrypted sensitive information is exposed.
Benefits of Private LLMs:
The adoption of private LLMs offers several advantages:
Enhanced Privacy: Users can harness the capabilities of LLMs without compromising their data, ensuring that sensitive information remains secure.
Building User Trust and Adoption: Prioritizing data privacy instills greater user confidence in AI technologies, encouraging wider adoption without privacy concerns.
Regulatory Compliance: Private LLMs align with evolving data protection regulations, ensuring organizations' compliance with data privacy laws.
Conclusion:
Private Large Language Models represent a critical step forward in reconciling the potential of AI with the paramount importance of data privacy. By incorporating innovative techniques like federated learning, differential privacy, and encrypted computation, developers can offer users the benefits of LLMs while upholding the highest standards of data security. As the field of AI continues to evolve, responsible Large Language Model Development Companies and adoption.